The Holy Grail for Location (GNSS) Manipulation Detection – Expert Interviews
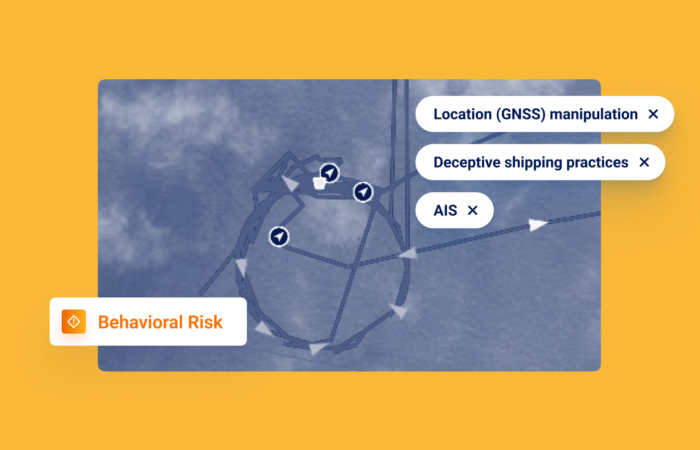
What’s inside?
Deep learning technology was recently integrated into Windward’s Location (GNSS) Manipulation Risk model, so our customers can stay on top of this morphing deceptive shipping practice (DSP). We sat down with three of the employees leading this new initiative:
- Dror Salzman, Windward’s Director of Trading & Shipping Products
- Tal Yogev, a Windward Data Scientist
- Ruth Ben Chaim, a Data Analyst at Windward
Click here to check out the interview with Dror.
Read on to learn from our top industry experts about the difficulties in moving from typologies to deep learning, the importance of a human-in-the-loop, and the “holy grail for location (GNSS) manipulation detection.”
“Detect Subtle Nuances that are Invisible to the Naked Eye.”
Tal Yogev, a Windward data scientist who is leading the development of this new technology integration, explains the difficulties involved in transitioning from a typology-based model to a deep learning model.
- Q: What are the challenges in going from a six-topologies model to deep learning?
Tal: There were a lot of challenges! (Laughs). The deep learning model, it learns how to find what you teach it to find. Most of what we had available to teach is the stuff from the existing typology models. If you do it naively, you are creating a deep learning model that mimics the typology models.
So the challenge was leveraging the additional technology that we are bringing to the table with additional domain expertise, which requires domain investigation to bring new types of cases that weren’t originally caught, to teach the deep learning model.
Windward experts look at the platform day in and day out. They know how vessels behave, when they see something suspicious, they flag it.
- Q: What are some additional challenges?
Tal: The data is very challenging, especially because we are trying to determine whether the transmission itself is real or fake – we want our data to be as clean as possible. We clean the raw AIS data with our patented process for fusion. That’s a huge advantage that we have, because imagine modeling raw AIS. We’ve actually done it and the signal is incredibly noisy.
Also, when we do a rule-based method, then we understand a subset of what bad guys can do. We define it, with the pros and cons of that. But now that we are training a deep learning model, it is more critical to clean the raw data to improve quality, as input for our model. We also have to have high quality labeled data, which Windward has because we have our internal team of experts and years of GNSS manipulation tech in production.
The raw data is just the AIS transmissions. The labels mean this vessel started manipulating on this date and time, and ended at this date and time. And then I can cut that, give those transmissions to the model and say, “This was a manipulation.”
I can then take a different set of times, give it to the deep learning model and say, “That same vessel did not manipulate here.” So the model can learn to differentiate.
Dror added: “There is a basic amount of label data that you must have to do a deep learning model. We are the only ones in the market currently who have enough label data on GNSS manipulations to be able to create a deep learning model.”
- Q: What is unique about this deep learning model?
Tal: It’s a different neural network architecture than our other models. It’s a neural network architecture that is common in the world of AI and we adapted it in collaboration with our domain experts to create a model that uses maritime signals that every maritime professional understands.
It puts a lot of signals together, with many examples, into a deep learning model. This model allows the model to detect subtle nuances that are invisible to the naked eye. And we also check it, using things like satellite imagery or manual inspection of the raw blips and other methods.
A lot of people can get AIS data. We combine them all with the fusion process. As in every data science project, quality data is fundamental and we wouldn’t expect this process to succeed without it.
“The Most Expert Person in This Domain in the Entire World.”
Dror describes Ruth Ben Chaim, a data analyst at Windward, “as the most expert person in this domain in the entire world. She’s seen the most cases.”
Ruth and her team are the “humans-in-the-loop” charged with checking every instance of location (GNSS) manipulation flagged by Windward’s Maritime AI™ platform, or raised by our customers and industry stakeholders.
- Q: What has your role been in terms of GNSS and the development of this new model?
Ruth: My main job was to correctly and accurately label the manipulations, because it’s so important for the model to get the correct label – the exact start and end date. If we give it inaccurate data, it will reduce its accuracy.
If you are lazy and say the manipulation started today and just default to midnight, but in reality, the vessel didn’t start manipulating until 6 a.m., now you have many incorrect hours influencing the model’s training. This is why a human-in-the-loop is so important.
Tal adds: “She saw many examples and helped point the research in the right direction. ‘These are the cases we are missing, we need to strengthen this area, we always are good at this.’ She helped check whether the results we see are reflected in the output that the customer is getting at the end of the day.”
- Q: How can Windward be so confident that it is right about the cases it flags?
Ruth: We have very high confidence in the GNSS cases we present. If we say it’s a GNSS case it is, because we know exactly when they start and end. We are able to review every single case, because our technology is so accurate. Too many false positives means you wouldn’t be able to go over everything.
- Q: How long does each check take? What are you looking for, specifically?
Ruth: The manipulations are getting harder and smarter. The ‘flower-shaped’ ones (ridiculous patterns) were easy. As the offenders get better, it’s harder to detect. Each check takes between 10 minutes to half an hour, depending on the difficulty of the case. Saying “yes” or “no” is easier than saying when the manipulation started and ended, which is critical for the deep learning model to keep training itself on accurate data.
- Q: Does GPS jamming in hot spots like the Middle East make location manipulation harder to detect?
Ruth: Expertise is important, because what’s happening in places like Lebanon now makes detection more difficult. Windward has the expertise, we understand the domain, this is an area that hardly has any GNSS cases, we know what’s happening in Lebanon now (jamming that is affecting all vessels), so we can make accurate determinations.
“The Holy Grail”
We gave Tal the last word…
1. Q: What is the next evolution planned for location (GNSS) manipulation detection?
Tal: I think the next major one is, the holy grail, is for it to go into auto-pilot mode. The idea is to get out of the cat-and-mouse game. We want to always stay ahead of the game.
Every time there was a case we missed, we’d ask, “Do we have to tweak the threshold and fix it by hand? Or create a new typology?” We are hoping to get to the point where there is a model, it produces outputs, we are verifying the outputs, and that is added to the dataset that the model will get retrained on regularly. As the world changes, the model also changes and improves. It would be training itself!