Deep Dive on Deep Learning for Deep Sea: Part 1
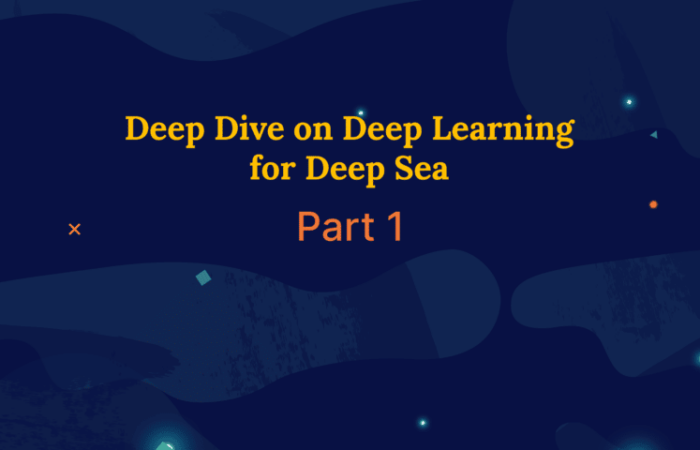
What’s inside?
By Shahar Margulis, R&D Data Analyst, Windward
Windward’s co-founder and CEO, Ami Daniel, likes to note that a decade ago we couldn’t even pick up where a vessel was on the open sea, but now we are seeing the emergence of a real maritime technology market. As the market matures, organizations throughout the maritime ecosystem are considering artificial intelligence (AI), machine learning, and deep learning solutions.
It can be difficult to properly evaluate and make the right decision, though, due to the overlap between these terms and the fact that some people seem to be using them differently. It might be helpful to take a step back and clarify, to establish a common language and understanding of these concepts and terms. After that, we’ll take a quick look at whether organizations looking for AI solutions for the maritime sphere should look for vendors with maritime expertise…
Let’s dive in…
Learning about AI, Machine Learning, & Deep Learning
Artificial intelligence (AI) broadly refers to a machine or system displaying and mimicking human-like behavior, such as learning and problem-solving. Machine learning is a subset of AI, and deep learning is a subset of machine learning. Both machine learning and deep learning use AI algorithms to make predictions or classifications using input data.
Machine learning enables intelligent systems to self-learn and constantly improve from data. The algorithms are fed with historical data, which helps them process and identify patterns automatically and make predictions about the future. They imitate the manner in which humans learn, but are able to perform tasks much more accurately, saving time and money. Machine learning algorithms process smaller amounts of data than deep learning algorithms, but are more susceptible to errors. It is important that the data sets are big enough to prevent biased predictions.
Deep learning is the sophisticated and mathematically complex evolution of machine learning, where computers learn to think and mimic the human brain using artificial neural networks (a logical structure of algorithms modeled on the brain). These neural networks enable the processing of various data types, such as documents, images, text, and audio. Deep learning models perform best when solving extremely complex problems and using high volumes of data, quickly turning it into actionable information.
Of these three methods, deep learning is most applicable to the maritime ecosystem, since each individual vessel generates large amounts of data, and the problems are certainly complicated and complex. Deep learning does require technical expertise.
What is Maritime AI™?
Maritime AITM is the result of training deep learning AI models to understand the multitude of factors affecting the maritime industry. These AI prediction algorithms weigh each factor, including all of those affecting container shipping. The data used to train these AI models comes from multiple sources, including AIS transmissions, weather forecasts, and historical data. A multi-source data pool helps deliver a real-time, accurate view of what is happening at sea, increasing efficiency and scalability by streamlining data collection and analysis processes, all while minimizing the need for any manual work.
AI Is Great, But Not Enough
Claiming that your solution is based on AI is quickly becoming a trend in the field of supply chain visibility. Without maritime experts and expertise, such solutions simply cannot provide the level of accuracy and consistency that is so challenging to reach. The main reason for this is that the ocean is such a complex landscape, in which a multitude of factors can affect a voyage from point A to point B, including human factors, force majeure, technical issues, unions, regulations, and many more. AI models must be trained and supervised by maritime industry experts who intimately understand the intricate nature of the ecosystem. As an example, only someone who has been in the industry long enough can begin to understand the chain of events and the thought process that can bring a ship captain to skip a port where he/she was scheduled to berth, and what could consequently be the possible destiny of the containers that were supposed to be unloaded there. That understanding can be transferred to a deep learning algorithm and then trained to identify and predict such behaviors.
Windward’s Ocean Freight Visibility solution leverages both maritime and AI expertise to train deep learning models to fuse huge amounts of data from multiple sources that are relevant to the supply chain, including AIS, port congestions and efficiency, vessel historical behaviors at the individual level, weather reports and forecasts, and more. This results in the most accurate real-time insights into any container’s voyage and predictions of its expected time of arrival (ETA) at the port of discharge (POD).
Look out for our next blog in this series, where we’ll discuss Windward’s ETA prediction solution in more detail and why we specifically chose deep learning for this model…